4. Ensembles
4.1. Ensemble reduction
This tutorial will explore ensemble reduction (also known as ensemble selection) using xscen
. This will use pre-computed annual mean temperatures from xclim.testing
.
[1]:
from xclim.testing import open_dataset
import xscen as xs
datasets = {
"ACCESS": "EnsembleStats/BCCAQv2+ANUSPLIN300_ACCESS1-0_historical+rcp45_r1i1p1_1950-2100_tg_mean_YS.nc",
"BNU-ESM": "EnsembleStats/BCCAQv2+ANUSPLIN300_BNU-ESM_historical+rcp45_r1i1p1_1950-2100_tg_mean_YS.nc",
"CCSM4-r1": "EnsembleStats/BCCAQv2+ANUSPLIN300_CCSM4_historical+rcp45_r1i1p1_1950-2100_tg_mean_YS.nc",
"CCSM4-r2": "EnsembleStats/BCCAQv2+ANUSPLIN300_CCSM4_historical+rcp45_r2i1p1_1950-2100_tg_mean_YS.nc",
"CNRM-CM5": "EnsembleStats/BCCAQv2+ANUSPLIN300_CNRM-CM5_historical+rcp45_r1i1p1_1970-2050_tg_mean_YS.nc",
}
for d in datasets:
ds = open_dataset(datasets[d]).isel(lon=slice(0, 4), lat=slice(0, 4))
ds = xs.climatological_mean(ds, window=30, periods=[[1981, 2010], [2021, 2050]])
datasets[d] = xs.compute_deltas(ds, reference_horizon="1981-2010")
datasets[d].attrs["cat:id"] = d # Required by build_reduction_data
datasets[d].attrs["cat:xrfreq"] = "AS-JAN"
/home/docs/checkouts/readthedocs.org/user_builds/xscen/conda/v0.8.2/lib/python3.11/site-packages/clisops/core/regrid.py:42: UserWarning: xarray version >= 2023.03.0 is not supported for regridding operations with cf-time indexed arrays. Please use xarray version < 2023.03.0. For more information, see: https://github.com/pydata/xarray/issues/7794.
warnings.warn(
ERROR 1: PROJ: proj_create_from_database: Open of /home/docs/checkouts/readthedocs.org/user_builds/xscen/conda/v0.8.2/share/proj failed
INFO:xclim:Fetching remote file: EnsembleStats/BCCAQv2+ANUSPLIN300_ACCESS1-0_historical+rcp45_r1i1p1_1950-2100_tg_mean_YS.nc
INFO:xclim:Fetching remote file md5: EnsembleStats/BCCAQv2+ANUSPLIN300_ACCESS1-0_historical+rcp45_r1i1p1_1950-2100_tg_mean_YS.nc.md5
/home/docs/checkouts/readthedocs.org/user_builds/xscen/conda/v0.8.2/lib/python3.11/site-packages/xscen/aggregate.py:90: FutureWarning: xs.climatological_mean is deprecated and will be abandoned in a future release. Use xs.climatological_op with option op=='mean' instead.
INFO:xclim:Fetching remote file: EnsembleStats/BCCAQv2+ANUSPLIN300_BNU-ESM_historical+rcp45_r1i1p1_1950-2100_tg_mean_YS.nc
INFO:xclim:Fetching remote file md5: EnsembleStats/BCCAQv2+ANUSPLIN300_BNU-ESM_historical+rcp45_r1i1p1_1950-2100_tg_mean_YS.nc.md5
/home/docs/checkouts/readthedocs.org/user_builds/xscen/conda/v0.8.2/lib/python3.11/site-packages/xscen/aggregate.py:90: FutureWarning: xs.climatological_mean is deprecated and will be abandoned in a future release. Use xs.climatological_op with option op=='mean' instead.
INFO:xclim:Fetching remote file: EnsembleStats/BCCAQv2+ANUSPLIN300_CCSM4_historical+rcp45_r1i1p1_1950-2100_tg_mean_YS.nc
INFO:xclim:Fetching remote file md5: EnsembleStats/BCCAQv2+ANUSPLIN300_CCSM4_historical+rcp45_r1i1p1_1950-2100_tg_mean_YS.nc.md5
/home/docs/checkouts/readthedocs.org/user_builds/xscen/conda/v0.8.2/lib/python3.11/site-packages/xscen/aggregate.py:90: FutureWarning: xs.climatological_mean is deprecated and will be abandoned in a future release. Use xs.climatological_op with option op=='mean' instead.
INFO:xclim:Fetching remote file: EnsembleStats/BCCAQv2+ANUSPLIN300_CCSM4_historical+rcp45_r2i1p1_1950-2100_tg_mean_YS.nc
INFO:xclim:Fetching remote file md5: EnsembleStats/BCCAQv2+ANUSPLIN300_CCSM4_historical+rcp45_r2i1p1_1950-2100_tg_mean_YS.nc.md5
/home/docs/checkouts/readthedocs.org/user_builds/xscen/conda/v0.8.2/lib/python3.11/site-packages/xscen/aggregate.py:90: FutureWarning: xs.climatological_mean is deprecated and will be abandoned in a future release. Use xs.climatological_op with option op=='mean' instead.
INFO:xclim:Fetching remote file: EnsembleStats/BCCAQv2+ANUSPLIN300_CNRM-CM5_historical+rcp45_r1i1p1_1970-2050_tg_mean_YS.nc
INFO:xclim:Fetching remote file md5: EnsembleStats/BCCAQv2+ANUSPLIN300_CNRM-CM5_historical+rcp45_r1i1p1_1970-2050_tg_mean_YS.nc.md5
/home/docs/checkouts/readthedocs.org/user_builds/xscen/conda/v0.8.2/lib/python3.11/site-packages/xscen/aggregate.py:90: FutureWarning: xs.climatological_mean is deprecated and will be abandoned in a future release. Use xs.climatological_op with option op=='mean' instead.
4.1.1. Preparing the data
Ensemble reduction is built upon climate indicators that are relevant to represent the ensemble’s variability for a given application. In this case, we’ll use the mean temperature delta between 2021-2050 and 1981-2010.
However, the functions implemented in xclim.ensembles._reduce
require a very specific 2-D DataArray of dimensions “realization” and “criteria”. That means that all the variables need to be combined and renamed, and that all dimensions need to be stacked together.
xs.build_reduction_data
can be used to prepare the data for ensemble reduction. Its arguments are:
datasets
(dict, list)xrfreqs
are the unique frequencies of the indicators.horizons
is used to instruct on which horizon(s) to build the data from.
Because a simulation could have multiple datasets (in the case of multiple frequencies), an attempt will be made to decipher the ID and frequency from the metadata.
[2]:
data = xs.build_reduction_data(
datasets=datasets,
xrfreqs=["AS-JAN"],
horizons=["2021-2050"],
)
data
/home/docs/checkouts/readthedocs.org/user_builds/xscen/conda/v0.8.2/lib/python3.11/site-packages/xscen/reduce.py:140: FutureWarning: updating coordinate 'criteria' with a PandasMultiIndex would leave the multi-index level coordinates ['lat', 'lon', 'time'] in an inconsistent state. This will raise an error in the future. Use `.drop_vars(['criteria', 'lat', 'lon', 'time'])` before assigning new coordinate values.
[2]:
<xarray.DataArray 'values' (realization: 5, criteria: 16)> array([[1.6385803, 1.6387024, 1.6386108, 1.6383972, 1.6400146, 1.6401978, 1.6411133, 1.6411743, 1.6436768, 1.6435242, 1.6429443, 1.6429138, 1.6479797, 1.6470337, 1.6461182, 1.6454468], [2.0663452, 2.0625305, 2.059143 , 2.056244 , 2.0645447, 2.0613098, 2.0582886, 2.0549316, 2.0631104, 2.0596619, 2.056549 , 2.0528564, 2.0624084, 2.0589905, 2.055359 , 2.0518494], [1.6872864, 1.690033 , 1.6922913, 1.6943359, 1.6863403, 1.689331 , 1.6921997, 1.6947937, 1.685852 , 1.6888733, 1.6918335, 1.6954346, 1.6836548, 1.6868286, 1.6904602, 1.6944885], [1.6043091, 1.6021423, 1.6007996, 1.5988159, 1.6131592, 1.611084 , 1.6099854, 1.6082764, 1.6217651, 1.620697 , 1.6195679, 1.618042 , 1.6308289, 1.6300964, 1.629364 , 1.62854 ], [1.3090515, 1.3095093, 1.3098145, 1.3091736, 1.3128052, 1.3133545, 1.3131714, 1.3131714, 1.3164062, 1.3165588, 1.316864 , 1.3173828, 1.3200073, 1.3203735, 1.3208923, 1.3215027]], dtype=float32) Coordinates: * realization (realization) <U8 'ACCESS' 'BNU-ESM' ... 'CCSM4-r2' 'CNRM-CM5' * criteria (criteria) int64 0 1 2 3 4 5 6 7 8 9 10 11 12 13 14 15 Attributes: long_name: criteria for ensemble selection
The number of criteria corresponds to: indicators x horizons x longitude x latitude
, but criteria that are purely NaN across all realizations are removed.
Note that xs.spatial_mean
could have been used prior to calling that function to remove the spatial dimensions.
4.1.2. Selecting a reduced ensemble
NOTE
Ensemble reduction in xscen
is built upon xclim.ensembles
. For more information on basic usage and available methods, please consult their documentation.
Ensemble reduction through xscen.reduce_ensemble
consists in a simple call to xclim
. The arguments are: - data
, which is the 2D DataArray that is created by using xs.build_reduction_data
. - method
is either kkz
or kmeans
. See the link above for further details on each technique. - kwargs
is a dictionary of arguments to send to the method chosen.
[3]:
selected, clusters, fig_data = xs.reduce_ensemble(
data=data, method="kmeans", kwargs={"method": {"n_clusters": 3}}
)
The method always returns 3 outputs (selected, clusters, fig_data): - selected
is a DataArray of dimension ‘realization’ listing the selected simulations. - clusters
(kmeans only) groups every realization in their respective clusters in a python dictionary. - fig_data
(kmeans only) can be used to call xclim.ensembles.plot_rsqprofile(fig_data)
[4]:
selected
[4]:
<xarray.DataArray 'realization' (realization: 3)> array(['ACCESS', 'BNU-ESM', 'CNRM-CM5'], dtype='<U8') Coordinates: * realization (realization) <U8 'ACCESS' 'BNU-ESM' 'CNRM-CM5' Attributes: axis: E
[5]:
# To see the clusters in more details
clusters
[5]:
{0: <xarray.DataArray 'realization' (realization: 1)>
array(['BNU-ESM'], dtype='<U8')
Coordinates:
* realization (realization) <U8 'BNU-ESM'
Attributes:
axis: E,
1: <xarray.DataArray 'realization' (realization: 3)>
array(['ACCESS', 'CCSM4-r1', 'CCSM4-r2'], dtype='<U8')
Coordinates:
* realization (realization) <U8 'ACCESS' 'CCSM4-r1' 'CCSM4-r2'
Attributes:
axis: E,
2: <xarray.DataArray 'realization' (realization: 1)>
array(['CNRM-CM5'], dtype='<U8')
Coordinates:
* realization (realization) <U8 'CNRM-CM5'
Attributes:
axis: E}
[6]:
from xclim.ensembles import plot_rsqprofile
plot_rsqprofile(fig_data)
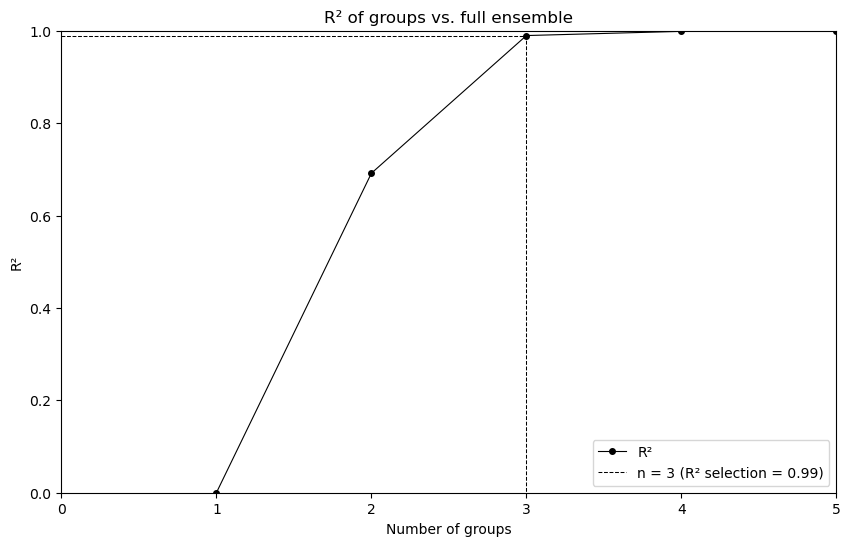
4.2. Ensemble partition
This tutorial will show how to use xscen to create the input for xclim partition functions.
[7]:
# Get catalog
from pathlib import Path
output_folder = Path().absolute() / "_data"
cat = xs.DataCatalog(str(output_folder / "tutorial-catalog.json"))
# create a dictionnary of datasets wanted for the partition
input_dict = cat.search(variable="tas", member="r1i1p1f1").to_dataset_dict(
xarray_open_kwargs={"engine": "h5netcdf"}
)
--> The keys in the returned dictionary of datasets are constructed as follows:
'id.domain.processing_level.xrfreq'
From a dictionary of datasets, the function creates a dataset with new dimensions in partition_dim
(["source", "experiment", "bias_adjust_project"]
, if they exist). In this toy example, we only have different experiments. - By default, it translates the xscen vocabulary (eg. experiment
) to the xclim partition vocabulary (eg. scenario
). It is possible to pass rename_dict
to rename the dimensions with other names. - If the inputs are not on the same grid, they can be regridded
through regrid_kw
or subset to a point through subset_kw
. The functions assumes that if there are different bias_adjust_project
, they will be on different grids (with all source
on the same grid). If there is one or less bias_adjust_project
, the assumption is thatsource
have different grids. - You can also compute indicators on the data if the input is daily. This can be especially useful when the daily input data is on different calendars.
[8]:
# build a single dataset
import xclim as xc
ds = xs.ensembles.build_partition_data(
input_dict,
subset_kw=dict(name="mtl", method="gridpoint", lat=[45.5], lon=[-73.6]),
indicators_kw={"indicators": [xc.atmos.tg_mean]},
)
ds
INFO:xscen.indicators:Computing 1 indicators.
INFO:xscen.indicators:1 - Computing tg_mean.
INFO:flox:Entering _validate_reindex: reindex is None
INFO:flox:Leaving _validate_reindex: reindex is False
INFO:flox:_choose_engine: Choosing 'flox'
INFO:flox:find_group_cohorts: blockwise is preferred.
INFO:flox:Entering _validate_reindex: reindex is False
INFO:flox:Leaving _validate_reindex: reindex is False
INFO:flox:Entering _validate_reindex: reindex is None
INFO:flox:Leaving _validate_reindex: reindex is False
INFO:flox:_choose_engine: Choosing 'flox'
INFO:flox:find_group_cohorts: blockwise is preferred.
INFO:flox:Entering _validate_reindex: reindex is False
INFO:flox:Leaving _validate_reindex: reindex is False
INFO:flox:Entering _validate_reindex: reindex is None
INFO:flox:Leaving _validate_reindex: reindex is False
INFO:flox:_choose_engine: Choosing 'flox'
INFO:flox:find_group_cohorts: blockwise is preferred.
INFO:flox:Entering _validate_reindex: reindex is False
INFO:flox:Leaving _validate_reindex: reindex is False
INFO:flox:Entering _validate_reindex: reindex is None
INFO:flox:Leaving _validate_reindex: reindex is False
INFO:flox:_choose_engine: Choosing 'flox'
INFO:flox:find_group_cohorts: blockwise is preferred.
INFO:flox:Entering _validate_reindex: reindex is False
INFO:flox:Leaving _validate_reindex: reindex is False
INFO:xscen.indicators:Computing 1 indicators.
INFO:xscen.indicators:1 - Computing tg_mean.
INFO:flox:Entering _validate_reindex: reindex is None
INFO:flox:Leaving _validate_reindex: reindex is False
INFO:flox:_choose_engine: Choosing 'flox'
INFO:flox:find_group_cohorts: blockwise is preferred.
INFO:flox:Entering _validate_reindex: reindex is False
INFO:flox:Leaving _validate_reindex: reindex is False
INFO:flox:Entering _validate_reindex: reindex is None
INFO:flox:Leaving _validate_reindex: reindex is False
INFO:flox:_choose_engine: Choosing 'flox'
INFO:flox:find_group_cohorts: blockwise is preferred.
INFO:flox:Entering _validate_reindex: reindex is False
INFO:flox:Leaving _validate_reindex: reindex is False
INFO:flox:Entering _validate_reindex: reindex is None
INFO:flox:Leaving _validate_reindex: reindex is False
INFO:flox:_choose_engine: Choosing 'flox'
INFO:flox:find_group_cohorts: blockwise is preferred.
INFO:flox:Entering _validate_reindex: reindex is False
INFO:flox:Leaving _validate_reindex: reindex is False
INFO:flox:Entering _validate_reindex: reindex is None
INFO:flox:Leaving _validate_reindex: reindex is False
INFO:flox:_choose_engine: Choosing 'flox'
INFO:flox:find_group_cohorts: blockwise is preferred.
INFO:flox:Entering _validate_reindex: reindex is False
INFO:flox:Leaving _validate_reindex: reindex is False
INFO:xscen.indicators:Computing 1 indicators.
INFO:xscen.indicators:1 - Computing tg_mean.
INFO:flox:Entering _validate_reindex: reindex is None
INFO:flox:Leaving _validate_reindex: reindex is False
INFO:flox:_choose_engine: Choosing 'flox'
INFO:flox:find_group_cohorts: blockwise is preferred.
INFO:flox:Entering _validate_reindex: reindex is False
INFO:flox:Leaving _validate_reindex: reindex is False
INFO:flox:Entering _validate_reindex: reindex is None
INFO:flox:Leaving _validate_reindex: reindex is False
INFO:flox:_choose_engine: Choosing 'flox'
INFO:flox:find_group_cohorts: blockwise is preferred.
INFO:flox:Entering _validate_reindex: reindex is False
INFO:flox:Leaving _validate_reindex: reindex is False
INFO:flox:Entering _validate_reindex: reindex is None
INFO:flox:Leaving _validate_reindex: reindex is False
INFO:flox:_choose_engine: Choosing 'flox'
INFO:flox:find_group_cohorts: blockwise is preferred.
INFO:flox:Entering _validate_reindex: reindex is False
INFO:flox:Leaving _validate_reindex: reindex is False
INFO:flox:Entering _validate_reindex: reindex is None
INFO:flox:Leaving _validate_reindex: reindex is False
INFO:flox:_choose_engine: Choosing 'flox'
INFO:flox:find_group_cohorts: blockwise is preferred.
INFO:flox:Entering _validate_reindex: reindex is False
INFO:flox:Leaving _validate_reindex: reindex is False
INFO:xscen.indicators:Computing 1 indicators.
INFO:xscen.indicators:1 - Computing tg_mean.
INFO:flox:Entering _validate_reindex: reindex is None
INFO:flox:Leaving _validate_reindex: reindex is False
INFO:flox:_choose_engine: Choosing 'flox'
INFO:flox:find_group_cohorts: blockwise is preferred.
INFO:flox:Entering _validate_reindex: reindex is False
INFO:flox:Leaving _validate_reindex: reindex is False
INFO:flox:Entering _validate_reindex: reindex is None
INFO:flox:Leaving _validate_reindex: reindex is False
INFO:flox:_choose_engine: Choosing 'flox'
INFO:flox:find_group_cohorts: blockwise is preferred.
INFO:flox:Entering _validate_reindex: reindex is False
INFO:flox:Leaving _validate_reindex: reindex is False
INFO:flox:Entering _validate_reindex: reindex is None
INFO:flox:Leaving _validate_reindex: reindex is False
INFO:flox:_choose_engine: Choosing 'flox'
INFO:flox:find_group_cohorts: blockwise is preferred.
INFO:flox:Entering _validate_reindex: reindex is False
INFO:flox:Leaving _validate_reindex: reindex is False
INFO:flox:Entering _validate_reindex: reindex is None
INFO:flox:Leaving _validate_reindex: reindex is False
INFO:flox:_choose_engine: Choosing 'flox'
INFO:flox:find_group_cohorts: blockwise is preferred.
INFO:flox:Entering _validate_reindex: reindex is False
INFO:flox:Leaving _validate_reindex: reindex is False
[8]:
<xarray.Dataset> Dimensions: (time: 2, scenario: 4, model: 1) Coordinates: * time (time) datetime64[ns] 2001-01-01 2002-01-01 * scenario (scenario) object 'ssp126' 'ssp245' 'ssp370' 'ssp585' * model (model) <U23 'NCC_NorESM2-MM_r1i1p1f1' lat float64 45.0 lon int64 -74 Data variables: tg_mean (scenario, model, time) float64 dask.array<chunksize=(4, 1, 2), meta=np.ndarray> Attributes: (12/22) comment: This is a test file created for the xscen tutori... version: v20191108 intake_esm_vars: ['tas'] cat:id: CMIP6_ScenarioMIP_NCC_NorESM2-MM_ssp245_r1i1p1f1... cat:type: simulation cat:processing_level: indicators ... ... cat:date_start: 2001-01-01 00:00:00 cat:date_end: 2002-12-31 00:00:00 cat:_data_format_: nc cat:path: /home/docs/checkouts/readthedocs.org/user_builds... intake_esm_dataset_key: CMIP6_ScenarioMIP_NCC_NorESM2-MM_ssp245_r1i1p1f1... history: [2024-02-12 21:51:12] gridpoint spatial subsetti...
Pass the input to an xclim partition function.
[10]:
# compute uncertainty partitionning
mean, uncertainties = xc.ensembles.hawkins_sutton(ds.tg_mean)
uncertainties
[10]:
<xarray.DataArray (uncertainty: 4, time: 4)> dask.array<concatenate, shape=(4, 4), dtype=float64, chunksize=(1, 2), chunktype=numpy.ndarray> Coordinates: lat float64 45.0 lon int64 -74 * time (time) object 2001-01-01 00:00:00 ... 2004-01-01 00:00:00 * uncertainty (uncertainty) object 'variability' 'model' 'scenario' 'total'
NOTE
Note that the figanos library provides a function fg.partition
to plot the uncertainties.